9.4: Bayesian statistics is to use Bayes theorem
Although there may be several opinions for the question
"What is Bayesian statistics?",
we think that
\begin{align}
\mbox{
Bayesian statistics is to use Bayes theorem
}
\end{align}
Thus,
Theorem 9.10 [The conditional probability]. Consider the mixed measurement ${\mathsf M}_{\overline{\mathcal A}} \big({\mathsf O}{{=}} $ $ (X \times Y , {\cal F} \boxtimes {\cal G} , H), $ $ {\overline S}_{[{}\ast] }(w) \big)$, which is formulated in the basic structure
\begin{align} [{\mathcal A} \subseteq \overline{\mathcal A} \subseteq B(H)] \end{align}Assume that a measured value $( x, y)$ $(\in X \times Y )$ is obtained by the mixed measurement${\mathsf M}_{\overline{\mathcal A}} \big({\mathsf O}{{=}} $ $ (X \times Y , {\cal F} \boxtimes {\cal G} , H), $ $ {\overline S}_{[{}\ast] }(w) \big)$ belongs to $ \Xi \times Y$ $(\in {\cal F})$. Then, the probability that $ y \in \Gamma $ is given by
\begin{align} \frac{{}_{\overline{\mathcal A}_*} (w, H(\Xi \times \Gamma))_{\overline{\mathcal A}} }{ {}_{\overline{\mathcal A}_*} (w, H(\Xi \times Y))_{\overline{\mathcal A}} } \quad (\forall \Gamma \in {\cal G}) \end{align}Proof This is due to the property (or, common sense) of conditional probability.
In the classical case, this is rewritten as follows.
Theorem 9.11 [Bayes' Theorem( in classical mixed measurement)]. Consider the simultaneous measurement ${\mathsf M}_{\overline{\mathcal A}} \big({\mathsf O}{{=}} $ $ (X \times Y , {\cal F} \boxtimes {\cal G} , F \times G), $ $ S_{[{}\ast] }({w_0}) \big)$ formulated in the classical basic structure $[C_0(\Omega ) \subseteq L^\infty(\Omega, \nu ) \subseteq B( L^2 (\Omega, \nu ) )]$. Here the observable ${\mathsf O}_{12}{{=}} (X \times Y , {\cal F} \boxtimes {\cal G} , F \times G)$ is defined by the simultaneous observable of the two observables ${\mathsf O}_{1}{{=}} (X , {\cal F} , F )$ and ${\mathsf O}_{2}{{=}} (Y , {\cal G} , G)$. That is,
\begin{align} (F \times G)(\Xi \times \Gamma ) = F(\Xi ) \cdot G(\Gamma) \qquad (\forall \Xi \in {\mathcal F}, \forall \Gamma \in {\mathcal G}) \tag{9.10} \end{align} Assume that$(a):$ | a measured value $( x, y)$ $(\in X \times Y )$ obtained by the mixed measurement ${\mathsf M}_{ L^\infty (\Omega ) } \big({\mathsf O}_{12}{{=}} $ $ (X \times Y , {\cal F} \boxtimes {\cal G} , F \times G), $ $ S_{[{}\ast] }({w_0}) \big)$ belongs to $ \Xi \times Y$ $(\mbox{where},\Xi \in {\cal F})$. |
Then, the probability such that "$ y \in \Gamma $" is given by
\begin{align} \frac{{}_{L^1(\Omega )} ({w_0}, H(\Xi \times \Gamma))_{{L^\infty (\Omega ) }} }{ {}_{ L^1(\Omega ) } ({w_0}, H(\Xi \times Y))_{{L^\infty (\Omega )}} } & \Big( = \frac{ \int_\Omega [F(\Xi )](\omega) \cdot [G(\Gamma )](\omega ) \cdot {w_0}(\omega ) \nu(d \omega ) }{ \int_\Omega [F(\Xi )](\omega) \cdot {w_0}(\omega ) \nu(d \omega ) } \Big) \tag{9.11} \end{align} Here,putting$(b):$ | $w_{\rm new}(\omega )= \frac{[F(\Xi)](\omega ) \cdot {w_0}(\omega )}{\int_\Omega [F(\Xi)](\omega ) \cdot {w_0}(\omega ) \nu(d \omega )} $ $(\;\; \forall \omega \in \Omega ) $ |
Remark 9.12 [How to understand Bayes' Theorem] Bayes' theorem 9.11 is usually read as follows.
$(a'):$ | If a measured value $ x$ $(\in X)$ obtained by the mixed measurement ${\mathsf M}_{L^\infty (\Omega ) } \big({\mathsf O}_1{{=}} $ $ (X , {\cal F} , F ), $ $ S_{[{}\ast] }({w_0}) \big)$ belongs to $ \Xi $ $(\in {\cal F})$, then, the following state collapse happens: $$ \underset{\mbox{ pre-state}}{\fbox{${w_0}$}} \xrightarrow[\mbox{ $x \in \Xi$}]{} \underset{\mbox{ post-state}}{\fbox{$w_{\rm new}$}} $$ |
The above (d) superficially contradicts the linguistic interpretation, which says that
\begin{align} \mbox{ "a state never moves".} \end{align}In this sense, the above (d) (or, (d$'$)) (i.e., Bayes theorem) is convenient and makeshift.
Answer 9.13 [Bayes' Theorem (=Problem9.5 and the answer to (c$_2$))]
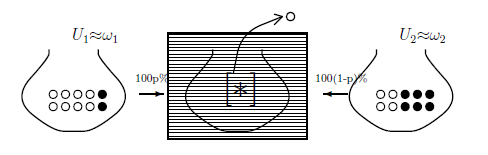
Asssume that the state space$\Omega=\{\omega_1, \omega_2\}$ is defined by the discrete metric with the following measure $\nu$:
\begin{align} \nu(\{ \omega_1 \})=1, \qquad \nu(\{ \omega_2 \})=1 \tag{9.13} \end{align}Thus, we start from the clasical basic structure:
\begin{align} [C_0(\Omega) \subseteq L^\infty (\Omega, \nu ) \subseteq B(L^2 (\Omega, \nu ))] \tag{9.14} \end{align}in which we consider the mixed measurement ${\mathsf M}_{{L^\infty (\Omega) }} ({\mathsf O} {{=}}$ $ ( \{ W,$ $ B \},$ $ 2^{\{ W, B \} } ,$ $ F) , S_{ [{}{\ast}]}{(w)})$. Here, the observable ${\mathsf O}_{WB} = ( \{ W, B \}, 2^{\{ W, B \} } , F_{WB})$ in $L^\infty (\Omega)$ is defined by
\begin{align} & [F_{WB}(\{ W \})](\omega_1)= 0.8, & \quad & [ F_{WB}(\{ B \})](\omega_1)= 0.2 \nonumber \\ & [F_{WB}(\{ W \})](\omega_2)= 0.4, & \quad & [F_{WB}(\{ B \})] (\omega_2)= 0.6 \tag{9.15} \end{align}Also,the mixed state $w_0 \in L^1_{+1}(\Omega , \nu )$ is defined by
\begin{align} w_0(\omega_1 ) = p, \qquad w_0(\omega_2)=1-p \tag{9.16} \end{align}Then, by Axiom$^{(m)}$1 ( in $\S$9.1), we see
$(a):$ | the probability that a measured value $x$ $(\in \{ W , B \})$ is obtained by ${\mathsf M}_{{L^\infty (\Omega) }} ({\mathsf O} {{=}}$ $ ( \{ W,$ $ B \},$ $ 2^{\{ W, B \} } ,$ $ F) , S_{ [{}{\ast}]}{(w)})$ is given by |
[$W^*$-algebraic answer to Problem 9.5(c$_2$) in $\S$9.1.2]
Since "white ball" is obtained by a mixed measurement ${\mathsf M}_{L^\infty (\Omega)}({\mathsf O}, S_{[*]}(w_0))$, a new mixed state $w_{\rm new} (\in L^1_{+1}(\Omega ))$ is given by
\begin{align} w_{\rm new} (\omega) & = \frac{ [F(\{ {{W}}\} )]( \omega) w_0 ( \omega)}{\int_\Omega [F( \{ {{W}}\} )](\omega) w_0(\omega) \nu(d \omega )} = \left\{\begin{array}{ll} \frac{\displaystyle 0.8 p }{\displaystyle 0.8p+0.4(1-p) } \qquad & (\mbox{when }\omega=\omega_1) \\ \\ \frac{\displaystyle 0.4(1- p) }{\displaystyle 0.8p+0.4(1-p) } \quad & (\mbox{when }\omega =\omega_2 ) \end{array}\right. \end{align}[$C^*$-algebraic answer to Problem 9.5(c$_2$)in $\S$9.1.2]
Since "white ball" is obtained by a mixed measurement ${\mathsf M}_{L^\infty (\Omega)}({\mathsf O}, S_{[*]}(\rho_0))$, a new {mixed state} $\rho_{\rm new} (\in {\mathcal M}_{+1}(\Omega ))$ is given by
\begin{align} \rho_{\rm new} & = \frac{ F(\{ {{W}}\} ) \rho_0 }{\int_\Omega [F( \{ {{W}}\} )](\omega) \rho_0(d \omega )} = \frac{\displaystyle 0.8 p }{\displaystyle 0.8p+0.4(1-p) } \delta_{\omega_1} + \frac{\displaystyle 0.4(1- p) }{\displaystyle 0.8p+0.4(1-p) } \delta_{\omega_2} \end{align}
Now、 you may ask What is Bayesian statistics? This is answered:
In most cases, it suffices to consider that